New AI innovations and applications are reaching consumers and businesses on an almost-daily basis. Building AI securely is a paramount concern, and we believe that Google’s Secure AI Framework (SAIF) can help chart a path for creating AI applications that users can trust. Today, we’re highlighting two new ways to make information about AI supply chain security universally discoverable and verifiable, so that AI can be created and used responsibly.
The first principle of SAIF is to ensure that the AI ecosystem has strong security foundations. In particular, the software supply chains for components specific to AI development, such as machine learning models, need to be secured against threats including model tampering, data poisoning, and the production of harmful content.
Even as machine learning and artificial intelligence continue to evolve rapidly, some solutions are now within reach of ML creators. We’re building on our prior work with the Open Source Security Foundation to show how ML model creators can and should protect against ML supply chain attacks by using SLSA and Sigstore.
Supply chain security for ML
For supply chain security of conventional software (software that does not use ML), we usually consider questions like:
- Who published the software? Are they trustworthy? Did they use safe practices?
- For open source software, what was the source code?
- What dependencies went into building that software?
- Could the software have been replaced by a tampered version following publication? Could this have occurred during build time?
All of these questions also apply to the hundreds of free ML models that are available for use on the internet. Using an ML model means trusting every part of it, just as you would any other piece of software. This includes concerns such as:
- Who published the model? Are they trustworthy? Did they use safe practices?
- For open source models, what was the training code?
- What datasets went into training that model?
- Could the model have been replaced by a tampered version following publication? Could this have occurred during training time?
We should treat tampering of ML models with the same severity as we treat injection of malware into conventional software. In fact, since models are programs, many allow the same types of arbitrary code execution exploits that are leveraged for attacks on conventional software. Furthermore, a tampered model could leak or steal data, cause harm from biases, or spread dangerous misinformation.
Inspection of an ML model is insufficient to determine whether bad behaviors were injected. This is similar to trying to reverse engineer an executable to identify malware. To protect supply chains at scale, we need to know how the model or software was created to answer the questions above.
Solutions for ML supply chain security
In recent years, we’ve seen how providing public and verifiable information about what happens during different stages of software development is an effective method of protecting conventional software against supply chain attacks. This supply chain transparency offers protection and insights with:
- Digital signatures, such as those from Sigstore, which allow users to verify that the software wasn’t tampered with or replaced
- Metadata such as SLSA provenance that tell us what’s in software and how it was built, allowing consumers to ensure license compatibility, identify known vulnerabilities, and detect more advanced threats
Together, these solutions help combat the enormous uptick in supply chain attacks that have turned every step in the software development lifecycle into a potential target for malicious activity.
We believe transparency throughout the development lifecycle will also help secure ML models, since ML model development follows a similar lifecycle as for regular software artifacts:
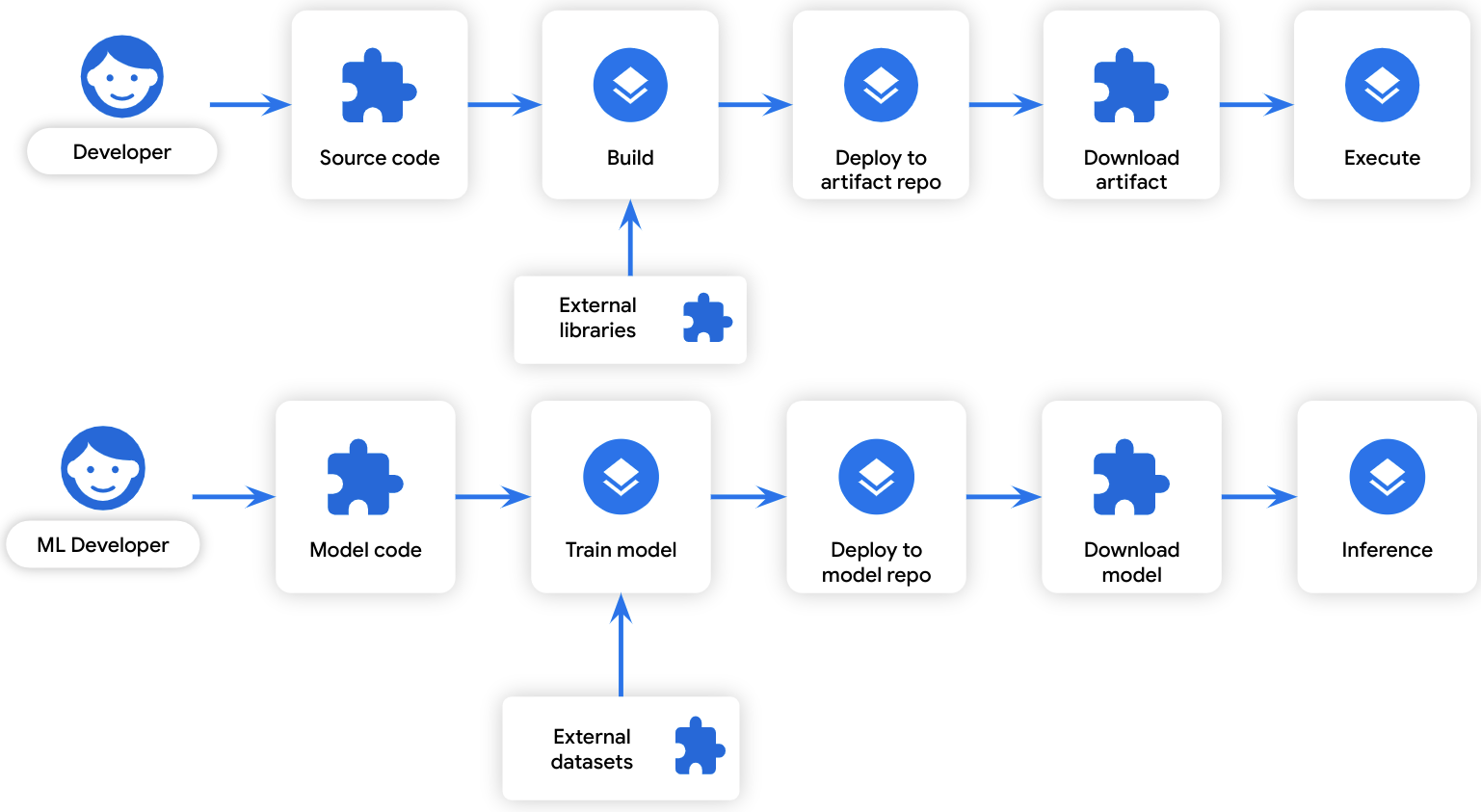
Similarities between software development and ML model development
An ML training process can be thought of as a “build:” it transforms some input data to some output data. Similarly, training data can be thought of as a “dependency:” it is data that is used during the build process. Because of the similarity in the development lifecycles, the same software supply chain attack vectors that threaten software development also apply to model development:
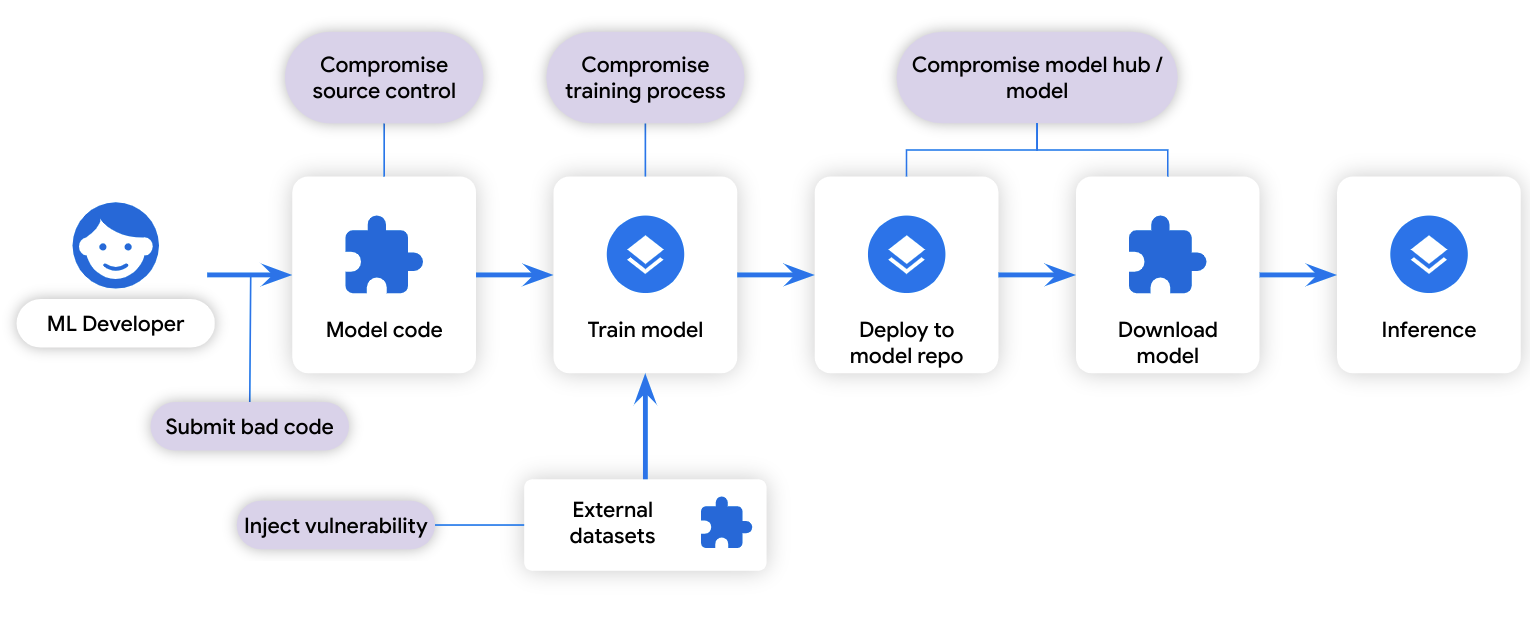
Attack vectors on ML through the lens of the ML supply chain
Based on the similarities in development lifecycle and threat vectors, we propose applying the same supply chain solutions from SLSA and Sigstore to ML models to similarly protect them against supply chain attacks.
Sigstore for ML models
Code signing is a critical step in supply chain security. It identifies the producer of a piece of software and prevents tampering after publication. But normally code signing is difficult to set up—producers need to manage and rotate keys, set up infrastructure for verification, and instruct consumers on how to verify. Often times secrets are also leaked since security is hard to get right during the process.
We suggest bypassing these challenges by using Sigstore, a collection of tools and services that make code signing secure and easy. Sigstore allows any software producer to sign their software by simply using an OpenID Connect token bound to either a workload or developer identity—all without the need to manage or rotate long-lived secrets.
So how would signing ML models benefit users? By signing models after training, we can assure users that they have the exact model that the builder (aka “trainer”) uploaded. Signing models discourages model hub owners from swapping models, addresses the issue of a model hub compromise, and can help prevent users from being tricked into using a bad model.
Model signatures make attacks similar to PoisonGPT detectable. The tampered models will either fail signature verification or can be directly traced back to the malicious actor. Our current work to encourage this industry standard includes:
- Having ML frameworks integrate signing and verification in the model save/load APIs
- Having ML model hubs add a badge to all signed models, thus guiding users towards signed models and incentivizing signatures from model developers
- Scaling model signing for LLMs
SLSA for ML Supply Chain Integrity
Signing with Sigstore provides users with confidence in the models that they are using, but it cannot answer every question they have about the model. SLSA goes a step further to provide more meaning behind those signatures.
SLSA (Supply-chain Levels for Software Artifacts) is a specification for describing how a software artifact was built. SLSA-enabled build platforms implement controls to prevent tampering and output signed provenance describing how the software artifact was produced, including all build inputs. This way, SLSA provides trustworthy metadata about what went into a software artifact.
Applying SLSA to ML could provide similar information about an ML model’s supply chain and address attack vectors not covered by model signing, such as compromised source control, compromised training process, and vulnerability injection. Our vision is to include specific ML information in a SLSA provenance file, which would help users spot an undertrained model or one trained on bad data. Upon detecting a vulnerability in an ML framework, users can quickly identify which models need to be retrained, thus reducing costs.
We don’t need special ML extensions for SLSA. Since an ML training process is a build (shown in the earlier diagram), we can apply the existing SLSA guidelines to ML training. The ML training process should be hardened against tampering and output provenance just like a conventional build process. More work on SLSA is needed to make it fully useful and applicable to ML, particularly around describing dependencies such as datasets and pretrained models. Most of these efforts will also benefit conventional software.
For models training on pipelines that do not require GPUs/TPUs, using an existing, SLSA-enabled build platform is a simple solution. For example, Google Cloud Build, GitHub Actions, or GitLab CI are all generally available SLSA-enabled build platforms. It is possible to run an ML training step on one of these platforms to make all of the built-in supply chain security features available to conventional software.
How to do model signing and SLSA for ML today
By incorporating supply chain security into the ML development lifecycle now, while the problem space is still unfolding, we can jumpstart work with the open source community to establish industry standards to solve pressing problems. This effort is already underway and available for testing.
Our repository of tooling for model signing and experimental SLSA provenance support for smaller ML models is available now. Our future ML framework and model hub integrations will be released in this repository as well.
We welcome collaboration with the ML community and are looking forward to reaching consensus on how to best integrate supply chain protection standards into existing tooling (such as Model Cards). If you have feedback or ideas, please feel free to open an issue and let us know.
Nessun commento :
Posta un commento